How Confidence Scoring Is Delivering Superior Cybersecurity Threat Analysis
- Cyber Jack
- Jul 11, 2023
- 3 min read
This guest blog was contributed by Bashyam Anant, Senior Director Product Management, Sumo Logic ML/AI and David Andrzejewski, Director Engineering, Sumo Logic ML / AI.
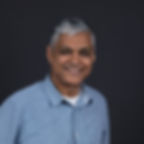
Security Operations Center (SOC) analysts must sift through a vast number of security events and alerts generated by their Cloud Security Information and Event Management (SIEM) system. Efficiently prioritizing and working through this backlog is crucial to delivering effective incident response and threat mitigation. Many SOC analysts successfully utilize confidence scores as a valuable tool in this process, and recent advancements in confidence scoring hold the promise of greater accuracy, insights, and utility for the SOC community.
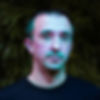
What Is Confidence Scoring?
Ideally, SOC analysts would inspect every SIEM-generated insight, then close each as either a true or false positive. In reality, the sheer number of insights makes this impractical.
Confidence scoring assigns a numerical value to security events or alerts based on their probability of being an actual security incident. Confidence scoring helps prioritize and focus on the most critical events or alerts, enabling analysts to allocate resources and respond to potential threats effectively.
Confidence scores can be crowdsourced in multi-tenant SIEM solutions. They are calculated via a machine learning algorithm that learns from the false and true positive resolutions of thousands of insights investigated by the population of SOC analysts using the SIEM. A confidence score is represented as a number from zero to one, with one representing relatively high confidence that the insight is a genuine compromise indicator.
The Benefits of Confidence Scoring
Incorporating the use of confidence scores offers security teams several benefits, including:
Risk-based prioritization. Confidence scores allow SOC analysts to focus attention and resources on high-confidence events, which are more likely to be legitimate threats or critical incidents.
Efficient resource allocation. Utilizing confidence scores helps quickly identify and deprioritize low-confidence or false positive alerts and reduce the number of alerts to investigate.
Continuous improvement. Analyzing confidence scores over time provides insights into the efficacy and performance of the SIEM system itself.
Help with auditing and compliance requirements. Confidence scores provide a valuable audit trail. This audit trail supports accountability, facilitates post-incident analysis, and helps demonstrate adherence to regulatory and compliance requirements.
Calibrating Confidence Scores
For confidence scores to be meaningful for prioritizing workloads, they must be interpretable as a probability that a given insight is a true positive. This is accomplished by calibrating confidence scores.
Calibration curves are a proven technique employed in machine learning (ML) to assess the quality and interpretability of the ML model when its results are used to drive an action.
For example, in this case, SOC analysts need the confidence score to represent the probability that a given insight is a true positive or a genuine compromise indicator worthy of investigation.
Capturing Thousands of Insights
Calibration curves are calculated by looking at thousands of insights over a historical period (e.g., 30 days) and assigning the insights to bins based on score ranges: 0 to 0.1, 0.1 to 0.2….., 0.9 to 1.0. In each bin, we can see the percentage of insights that are actual true positives. As illustrated by the graph below, for each bin, the x-axis is the midpoint of the bin range, while the y-axis is the share of true positives in the bin. If confidence scores are representative of probability, then we would expect the true positive ratio to be close to the dotted line.

The graph shows that the ML model in our example is well-calibrated at the extremes. For scores between 0.2 and 0.6, the calibration curve suggests that the confidence score might be overestimating the number of true positives.
In other words, the SOC analyst might be marking more false positives than are expected from a well-calibrated model. Either way, the calibration curve should lead analysts to prioritize investigating insights with scores greater than 0.6 and, time permitting, walk down the remaining insights sorted by confidence scores.
A Valuable Addition to Your SOC Analyst Toolkit
Confidence scoring can be an excellent tool for prioritizing your insights backlog by allowing you to focus on high-risk insights with high-confidence scores. Using confidence scores can reduce SOC analyst workload and burnout, improve the performance of the SIEM system over time, and create an audit trail that supports compliance. ###